ABSTRACT
The goal of the study was three-fold: to investigate medical student behaviors (e.g., changing answers) while taking high-stakes multiple-choice exams; to determine if specific behaviors were associated with performance on the exam; and to determine if there are associations of learning style, as measured by Kolb’s Learning Style Inventory (LSI), with test-taking activities. We developed high-stakes, on-line exam applications that included server event logs, which provided a time sequence of entries/activities that students made while taking their exam. This new paradigm allows collection of detailed test taking behaviors that can be used to test a variety of hypotheses. Test taking activities were extracted from the event logs for a mid-term anatomy exam given to freshman medical students. Although student exam-taking activities showed considerable variability, one notable finding was that when students changed answers, they were 3 times more likely (on average) to change their answer from incorrect-to-correct than they were to change it from correct-to-incorrect. Correlation of test-taking behaviors with performance on the exam revealed that there were significant negative correlations with the number of times answers were changed, and the number of times answers were changed from correct-to-incorrect. There were also significant associations of learning styles with exam-taking behaviors. The most consistent differences between students who did the best on the exam (Assimilators) and those who did the worst (Accommodators), concerned the frequencies with which answers were changed. Differences between Accommodators and Convergers, who received the next highest average score, involved behaviors related to variables other than changing answers (e.g., time spent reviewing the exam and number of questions marked for review). In summary, the use of objective computer entry logs allowed a better understanding of the associations of test-taking behaviors with academic performances and with learning styles. Based on these findings, learning strategies might be designed to help students cope with courses that rely heavily on multiple-choice exams for assessing student achievement.
INTRODUCTION
Computer technology is increasingly crucial to the training of physicians (cf.1). One area where computers are rapidly impacting training at all levels is their use in assessments, especially those involving multiple-choice exams. Although student attitudes to computerized testing are generally positive2, this new testing environment has raised concerns such as effects of computer anxiety and attitudes, sufficiency of resources, and reliability as compared to paper-and-pencil multiple-choice tests3-7.
Several studies have demonstrated associations between learning style and academic performance across the professional spectrum of medical training8-12 as well as in other disciplines13,14. More specifically, Lynch et al. 9 showed that medical students with a learning style preference of abstract conceptualization tended to perform better on the multiple-choice United States Medical Licensing exam (USMLE). This association between learning style and performance on multiple-choice exams requires a better understanding because of the broad implications both to the individual student progress and assessment in the medical curriculum. As computerized exams become more commonplace in the medical curriculum, possible effects of learning style on test-taking behaviors and performance on exams are more easily studied because of the ease with which objective data can be collected unobtrusively.
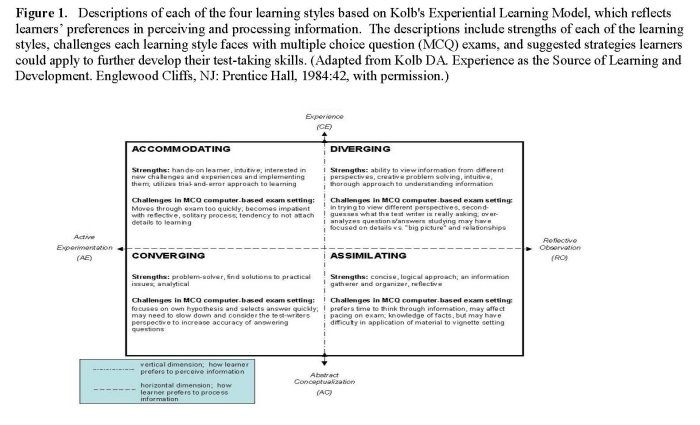
We undertook the present study as part of an initiative at the Stritch School of Medicine to develop web-based applications for delivery of high-stakes exams throughout the 4-year curriculum. The applications include server event logs of individual student actions while taking exams. These event logs were used to determine the association of test-taking behaviors with performance on the exam and with learning style as measured by Kolb’s Learning Style Inventory (LSI)15.
Kolb’s theory of learning style consists of four stages in the cycle of learning: concrete experience (CE), reflective observation (RO), abstract conceptualization (AC) and active experimentation (AE). These four stages represent two dimensions. CE and AC represent the vertical dimension of perceiving information. RO and AE represent the horizontal dimension of processing information. These two dimensions create four modes or styles of learning (Figure 1). The completed Learning Style Inventory (LSI) provides a score for each stage in the learning cycle. By calculating the results of the LSI and applying the scores to the Learning Style Type Grid, a learning style is defined based on each individual’s preference for how they perceive (CE-AC=perceiving score) and process information (RO-AE = processing score); thus, designating the quadrant that defines their learning style. The four modes or learning styles result from these dimensions: Diverging (CE/RO); Assimilating (RO/AC); Converging (AC/AE) Accommodating (AE/CE) (Figure 1).
The purpose of our study was three-fold: to investigate medical student behaviors (e.g., changing answers) while taking high-stakes multiple-choice exams; to determine if specific behaviors were associated with performance on the exam; and to determine if there are associations of learning style, as measured by Kolb’s Learning Style Inventory (LSI), with test-taking behaviors.
MATERIALS AND METHODS
Administration of Kolb’s Learning Style Inventory: The paper-based version of Kolb’s LSI (Version 3) was administered to the first year medical class (n=137) during orientation week. An overview of Kolb’s Model of Experiential Learning was presented to the students, followed by their completion and scoring of the inventory. The assessments were collected and calculations were verified and recorded. Additional small group workshops were given to the students, which provided a more in-depth discussion of learning style preferences, strengths and challenges. Administration of the LSI was coordinated through the Teaching and Learning Center at the Stritch School of Medicine (SSOM). The distribution of learning styles was as follows: Convergers (n=64); Accommodators (n=19); Divergers (n=15); and Assimilators (n=39).
On-Line Exam Application: The web-based exam database and applications were developed as part of the Loyola University Medical Education Network (LUMEN) to provide on-line exams. The exam interface (Figure 2) allows students to cross out answers, add notes, and submit answers for later review in the event they are unsure of the answer. The exam applications were constructed utilizing Allaire’s ColdFusion (v4.5) for middle-tier application development and Microsoft SQL Server (v7.0) for database services. Secure portal access is accomplished through the “myLUMEN” student portal, which uses Secure Socket Layer (SSL v3.0) with 128-bit digital certificates provided by Versign.
Administration of On-Line Exam: The exam analyzed for this study was the second midterm comprising 75 multiple-choice questions (see Figure 2 for an example of the questions). It was given to students (n=137) in the first year medical anatomy course. Students were assigned to specific computer stations in the learning laboratories and they accessed the exam through their secure student portal. They were given 2 hours to complete the exam.
Data Collection and Analysis: Server event logs for each student were exported into an Excel database. These logs included a timeline (by seconds) for all mouse click and keystroke activities from the time each student opened the exam to the time he/she clicked the “Finalize Exam” button. Each of the variables analyzed in this study (See Table 1) was manually extracted from the event logs. The total time spent taking the exam was the time from release of the exam to the time each student finalized his/her exam. The time spent reviewing the exam was estimated by subtracting the time when the last question was viewed from the total time of the exam. All data were entered into an Excel spreadsheet and the names of students deleted prior to further analyses in order to preserve anonymity. The data were analyzed using analysis of variance, Pearson correlation coefficients (after showing that the data were normally distributed), and the Kendall Coefficient of Concordance16. Given that eight tests of significance each needed to be conducted with the analysis of variance and Pearson coefficients, a Bonferroni adjusted p value of .0063 (.05/8) was used. No adjusted p value was used with the Kendall coefficients because only two such coefficients were computed.
The study was approved by the IRB.
RESULTS
Student behaviors while taking a multiple-choice exam. Table 1 illustrates the considerable variability in the activities of students while taking the exam. For instance, the total number of times students submitted answers for the 75 questions ranged from 76 to 266. The number of questions marked for review ranged between 0 and 67, and students spent an average of about 30 minutes reviewing their exam. The maximum number of answers changed was 21 (out of 75), with 99% (135/137) changing 1 or more answers. When students changed answers, they were 3 times more likely (on average) to change their answer from incorrect-to-correct than they were to change it from correct-to-incorrect.
Are exam-taking behaviors associated with performance on the exam? Pearson’s correlations were used to evaluate whether any of the variables measured were associated with performance on the exam. There were no significant correlations with time spent on the exam, time spent reviewing the exam, the number of answers submitted, nor the number of questions marked for review (Table 2). However, there were significant negative correlations with the number of times answers were changed, and the number of times answers were changed from correct-to-incorrect. Interestingly, there was no correlation between grade and the number of times answers were changed from incorrect-to-correct.
Are exam-taking behaviors associated with learning style? We were next interested in determining whether learning styles were associated with differences in exam activities. Results from the analysis of variance did not reveal any association of the Kolb learning styles when the data were sorted according to Kolb’s four learning styles with the variables listed in Tables 1-3 (p’s ranged from .268 to .842; data not shown). However, a 5% difference in grade between Assimilators (86.1) and Accommodators (81.1) as seen in Table 3 prompted us to consider the possibility that a combination of behaviors accounted for the differences in performance on the exam. Trends in the data were evident when the group averages were ranked (Table 3). The most consistent differences between students who did the best on the exam (Assimilators) and those who did the worst (Accommodators), concerned the frequencies with which answers were changed from incorrect-to-correct and vice-versa (Table 3). Differences between Accommodators and Convergers, who received the next highest average score, involved behaviors related to variables other than changing answers (e.g., time spent reviewing the exam and number of questions marked for review). Associations of learning style with test taking behaviors were tested using the Kendall Coefficient of Concordance test 16 using the rank orders. Learning styles were found to be significantly associated with answer changing (W = .91, x2 = 8.20, df = 3, p = .042) as well as with behaviors other than answer changing (W = .68, x2 = 10.20, df = 3, p = .017).
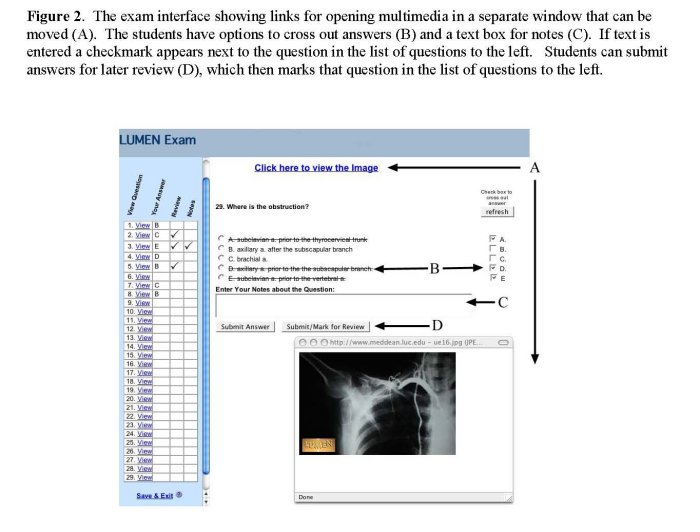
DISCUSSION
Our first goal to study student behaviors while taking an on-line exam illustrates an important advantage of server-based applications to collect objective data unobtrusively. A number of basic statistics can be useful for administration of future exams. For instance, knowing the average amount of time that students spend on individual questions can be used to better judge an appropriate time for the whole exam. Additionally, determining the frequency with which individual questions are marked for review can assist course directors and faculty in evaluating the appropriateness of the questions (e.g., is the question ambiguous).
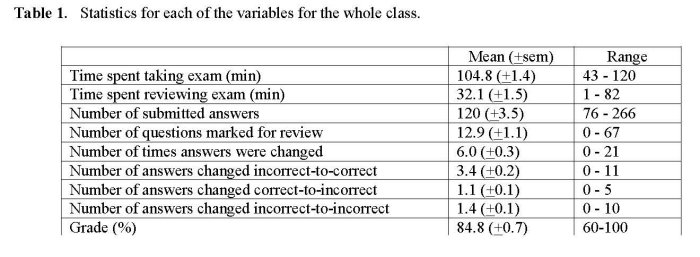
Our finding that students who change answers are more likely to change them from incorrect-to-correct is consistent with several other reports17-20. This tendency was more recently confirmed in a study using midterm results from an undergraduate psychology course where tabulations of erasure marks showed that 51% of changed answers were from wrong to right outnumbering changes from right to wrong by a factor of 2-to-121. The authors further showed that 54% of the students improved their grades by changing answers. Even more impressive were the present findings that 85% of the medical students improved their grade by changing answers; 5% made their grade worse; while the remaining 10% had no net change in grade.
A second goal of our study was to determine if specific behaviors were associated with performance on the exam. Although changing answers from wrong to right improved the score for most students in the present study, this activity was not correlated with grade on the exam. In fact, students in the lower quartile changed, on average, a slightly higher number of answers from incorrect to correct compared to students in the upper quartile (data not shown). In contrast, there were significant negative associations between grade and the frequency with which students changed their answers from right to wrong and from wrong to wrong. The overall increase in number of changed answers from those students who received a lower grade most likely reflects a general lack of confidence in their knowledge. Their greater tendency to change wrong answers to other wrong answers is certainly consistent with being unsure of their knowledge while taking the exam.
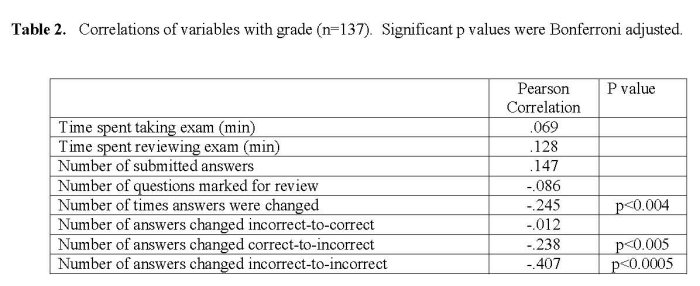
A third goal of the study was to examine if there were associations of exam-taking behaviors with specific learning styles. The wide variability in the behaviors associated with taking exams was not entirely unexpected in view of similarly large variability in medical student use of computer aided instructional resources22. Many of the factors explaining this variability have not been determined, however some possible associations with personality/learning styles have been investigated13,23,24. Results from the present study suggest that learning styles are associated with a combination of behaviors while taking multiple-choice exams. More specifically, Accommodators, who received the lowest average score on the exam, took the least amount of time for the exam, spent the least amount of time reviewing the exam, spent the least amount of time answering questions, had the fewest number of submitted answers, marked the fewest questions for review, changed the fewest answers from wrong-to-right, and changed the most answers from right-to-wrong.
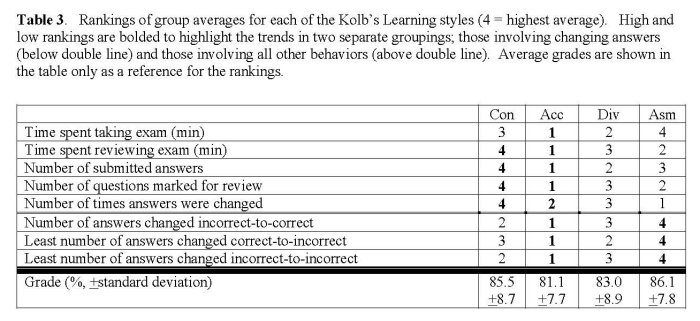
These findings are consistent with the Accommodating style, which reflects a learning preference for action and implementation (active experimentation – AE); thus, reducing the learner’s tendencies toward reflection and review (reflective observation – RO). Accommodators utilize an intuitive, trial-and-error approach to solving problems (concrete experience – CE) and in new learning situations, tend to rely heavily on others for information and details rather than their own analytic ability (abstract conceptualization – AC). Therefore, the stationary, non-interactive environment of the standardized exam setting is in direct conflict with the strengths that optimize and reflect the learning preferences of the Accommodating style.
Our study showed that Assimilators and Convergers had the best scores on the exam, which is consistent with other research showing that students with a preference for abstract conceptualization (AC) tended to perform better on multiple-choice exams9,10. The present results extend these findings by providing insights into specific activities and behaviors that are associated with performance on exams. Acquiring a better understanding of the association of learning styles with academic performances is important for those courses that rely heavily on multiple-choice exams for assessing student achievement. For the assessor, it can contribute to creation of an assessment tool that reflects a balance of diverse questions to challenge all learning styles in content and process. Probably more importantly, for the medical student, it can help them adapt their learning style preferences to optimize their test-taking strategies. Learning strategies might be designed to help students cope with a variety of examination settings. For example, Accommodators preparing for multiple-choice exams could practice organizing and analyzing their own information, identify patterns, build conceptual models, slow down and reflect before action. By utilizing an awareness of learning styles in correlation with exam behaviors and results, students can better prepare for the ongoing multiple-choice exams that the health profession requires. It is important for learners to not only set goals for development per their current learning style (see Figure 1), but to increase their awareness of strengths and challenges of other learning styles.
A noteworthy limitation of this study was sample size. We found that group sizes for the four Kolb learning styles only provided an average of 22% power for achieving statistical significance with analysis of variance at the .05 level given the magnitude of differences that were observed in the data. As the Kolb’s LSI is completed by future classes, data will be combined for analysis.
Having only a single examination was another limitation of our study and we are working to develop report applications, which will allow more efficient extraction of data from the event logs. When these are complete, we plan to extend our research to address a number of important questions that arise from this study. First, will intervention influence a student’s behavior on subsequent exams? Learning assistance offered to students having academic difficulties could include analyses of their activities during the exam, which may provide helpful insights into their test-taking skills allowing them to modify behaviors that are associated with poor performance as discussed above (see Figure 1). A second important question is whether individual exam behaviors are consistent from exam to exam and from course to course? The data collected for this study were from an exam in gross anatomy, which emphasizes factual knowledge. Would similar behaviors persist in a course requiring more conceptualizations? Based on significant correlations of individual performances from exam to exam in the anatomy course (unpublished observations), we predict that exam-taking behaviors are also consistent on exams within a course. Finally, to what degree do test-taking behaviors change over the curriculum and are these changes associated with changes in learning style that are known to occur with medical training25-27?
ACKNOWLEDGEMENTS
We gratefully acknowledge the following individuals who facilitated development and implementation of the applications for the LUMEN on-line exams (alphabetical order): Dr. A. Chandrasekhar, Dr. B. Espiritu, E. Fabro, G. Klitz, A. Hoyt, R. Naheedy, and R. Price.
REFERENCES
- Seago, B.L., Schlesinger, J.B., and Hampton, C.L. Using a decade of data on medical student computer literacy for strategic planning. Journal of Medical Library Associations. 2002; 90(2):202-9.
- Ogilvie, R.W., Trusk, T.C., and Blue, A.V. Student’s attitudes towards computer testing in a basic science course. Medical Education. 1999; 33:828-31.
- Clariana, R., and Wallace, P. Paper-based versus computer-based assessment: key factors associated with the test mode effect. British Journal Educational Technology. 2002; 33(5):593-602.
- Hahne, A.K., Benndorf, R., Frey, P., and Herzig, S. Attitude towards computer-based learning: determinants as revealed by a controlled interventional study. Medical Education. 2005; 39:935-43.
- Lee, G., and Weerakoon, P. The role of computer-aided assessment in health professional education: a comparison of student performance in computer-based and paper-and-pen multiple-choice tests. Medical Teaching. 2001; 23(2):152-7.
- Kreiter, C., Peterson., M.W., Ferguson, K., and Elliott, S. The effects of testing in shifts on a clinical in-course computerized exam. Medical Education. 2003; 37:202-4.
- Rozell, E.J., and Gardner, W.L.I. Cognitive, motivation, and affective processes associated with computer-related performance: a path analysis. Comparative Human Behavior. 2000; 16:199-2000.
- Markert, R.J. Learning style and medical students’ performance on objective examinations. Perception Motor Skills. 1986; 62(3):781-2.
- Lynch, T.G., Woelfl, N.N., Steele, D.J., and Hanssen, C.S. Learning style influences student examination performance. American Journal Surgery. 1998; 176(1):62-6.
- Newland, J.R., and Woelfl, N.N. Learning style inventory and academic performance of students in general pathology. Bulletin Pathology Education. 1992; 17:77-81.
- Chapman, D.M., and Calhoun, J.G. Validation of learning style measures: implications for medical education practice. Medical Education. 2006; 40:576-83.
- Smits, P.B.A., Verbeek, J.H.A.M., Nauta, M.C.E., Ten Cate, T.J., Metz, J.C.M., and Van Dijk, F.J.H. Factors predictive of successful learning in postgraduate medical education. Medical Education. 2004; 38:758-66.
- Cordell, B.J. A study of learning styles and computer-assisted instruction. Comparative Education. 1991; 16(2):175-83.
- Hamkins, G. Motivation and individual learning styles. Engineering Education. 1974; 64:408-11.
- Kolb, DA. Experiential Learning: Experience as the Source of Learning and Development. Englewood Cliffs, N.J.: Prentice-Hall, Inc. 1984.
- Siegel, S., and Castellan, N.J. Nonparametric statistics for the behavioral sciences. 2nd ed. New York: McGraw-Hill. 1988.
- Ferguson, K.J., Kreiter, C.D., Peterson, M.W., Rowat, J.A., and Elliott, S.T. Is that your final answer? Relationship of changed answers to overall performance on a computer-based medical school course examination. Teaching and Learning in Medicine. 2002; 14(1):20-3.
- Fischer, M.R., Herrmann, S., and Kopp, V. Answering multiple-choice questions in high-stakes medical examinations. Medical Education. 2005; 39:890-4.
- Range, L.M., Anderson, H.N., and Wesley, A.L. Personality correlates of multiple choice answer-changing patterns. Psychology Reports. 1982; 51:523-7.
- Vispoel, W.P. Reviewing and changing answers on computer-adaptive and self-adaptive vocabulary tests. Journal of Educational Measures. 1998; 35:328-45.
- Kruger, J., Wirtz, D., and Miller, D.T. Counterfactual thinking and the first instinct fallacy. Journal of Personality and Social Psychology. 2005; 88(5):725-35.
- McNulty, J.A., Halama, J., Daudzvardis, M.F., and Espiritu, B. Evaluation of web-based computer-aided instruction in a basic science course. Academic Medicine. 2000; 75(1):59-65.
- McNulty, J.A., Espiritu, B., and Halsey, M. Medical student use of computers is correlated with personality. Journal of the International Association of Medical Science Educators. 2002; 12:9-13.
- McNulty, J.A., Espiritu, B., Halsey, M., and Mendez, M. Personality preference influences medical student use of specific computer-aided instruction (CAI). BMC Medical Education. 2006; 6:7.
- Lindemann, R., Duek, J.L., and Wilkerson, L. A comparison of changes in dental students’ and medical students’ approaches to learning during professional training. European Journal of Dental Education. 2001; 5(4):162-7.
- Newble, D.I., and Gordon, M.I. The learning style of medical students. Medical Education. 1985; 19(1):3-8.
- Slotnick, H.B. How doctors learn: education and learning across the medical-school-to-practice trajectory. Academic Medicine. 2001; 76:1013-26.